Assumptions of Linear Regression Models
Post Date:
Quant in Practice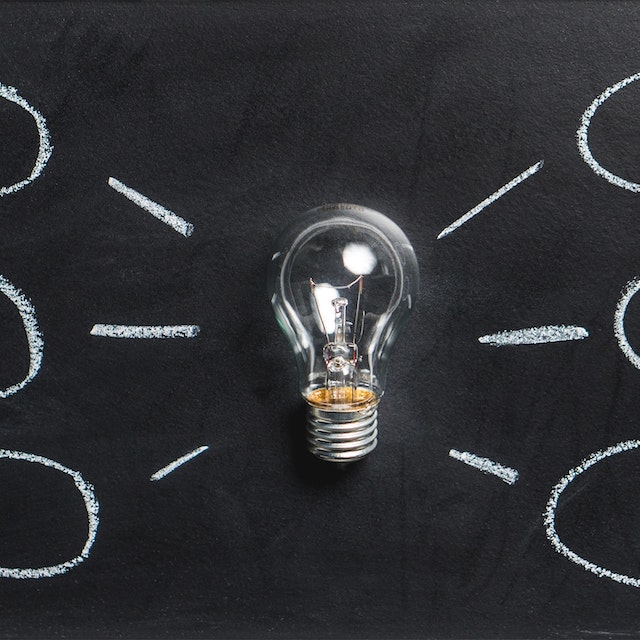
In statistics and data analysis, linear regression models stand as stalwarts, offering a powerful tool to unravel relationships between variables and make predictions. Whether employed in economics, social sciences, or natural sciences, these models provide a structured framework for understanding the dynamics of dependent and independent variables. However, the reliability and interpretability of linear regression models are contingent upon a set of crucial assumptions that underpin their functionality. This essay delves into the fundamental assumptions of linear regression models, shedding light on the bedrock upon which this statistical technique is built.
Assumption 1: Linearity
At the heart of linear regression lies the presumption of linearity, which posits that the relationship between the independent and dependent variables is linear. In simpler terms, this implies that any change in the independent variable(s) will yield a proportional and constant change in the dependent variable. If this assumption is violated, the model's predictive power is compromised, and alternative modelling approaches should be considered.
Assumption 2: Independence of Errors
To maintain the integrity of linear regression, the errors, or residuals, resulting from the model should be independent. In other words, the error of one observation should not be influenced by the error of another observation. This assumption is vital because correlated errors can lead to inefficient parameter estimates and inaccurate inferences.
Assumption 3: Homoscedasticity
Homoscedasticity, or the constant variance of errors, is another pivotal assumption. The spread of residuals should be consistent across all levels of the independent variables. When the variance of residuals systematically changes with the values of the independent variables, the model's reliability diminishes. Heteroscedasticity, or the violation of this assumption, could lead to biased coefficient estimates and unreliable hypothesis tests.
Assumption 4: Normality of Errors
The normality assumption stipulates that the errors follow a normal distribution. While this assumption is not critical for making predictions, it is essential for performing statistical inference, such as hypothesis testing and constructing confidence intervals. Deviations from normality can distort p-values and undermine the validity of the model.
Assumption 5: No Multicollinearity
Multicollinearity occurs when independent variables in the model are highly correlated. This assumption posits that there should be no perfect linear relationships between predictors. Multicollinearity can confound the interpretation of individual predictors and inflate standard errors, making it challenging to identify the unique contribution of each variable.
Assumption 6: No Endogeneity
Endogeneity implies that one or more independent variables are correlated with the error term. In other words, some omitted factors affect both the dependent and independent variables, leading to biased coefficient estimates. Detecting and addressing endogeneity is crucial for ensuring the model's validity.
These six assumptions collectively form the bedrock of linear regression models. Deviations from these assumptions can introduce bias, weaken the model's predictive power, and undermine the reliability of statistical inferences. Consequently, researchers and analysts must assess the validity of these assumptions when employing linear regression techniques.
In conclusion, the assumptions of linearity, independence of errors, homoscedasticity, normality of errors, no multicollinearity, and no endogeneity constitute the foundational principles upon which linear regression models rely. Understanding and validating these assumptions are indispensable steps in ensuring the efficacy and reliability of this widely used statistical tool. By adhering to these assumptions, analysts unlock the full potential of linear regression models to elucidate complex relationships and make informed predictions in various fields of study.
Last Update: June 2, 2025, 11:01 a.m.