Econometric Models: A Comprehensive Overview
Post Date:
Analysis Data Models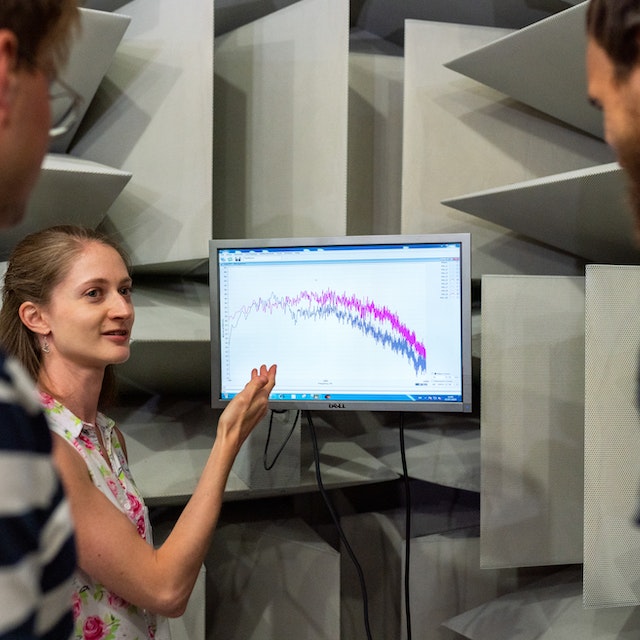
Econometric models are the cornerstone of modern economic analysis, allowing economists and policymakers to decipher the intricacies of economic phenomena, predict future trends, and formulate effective policies. These models are invaluable tools for examining the relationships between economic variables, estimating parameters, and testing hypotheses. In the realm of econometrics, a diverse array of models are tailored to address specific questions and scenarios. This essay embarks on a journey to explore some of the fundamental types of econometric models that shape economic understanding and decision-making.
Linear Regression Models
Linear regression models are the bedrock of econometrics. They are wielded to analyze the relationship between a dependent variable and one or more independent variables, assuming a linear functional form. Whether examining the impact of interest rates on investment or the relationship between income and consumption, linear regression models provide a clear framework for understanding how changes in one variable affect another. Furthermore, these models are versatile, accommodating various forms such as multiple regression, time series regression, and panel data regression.
Time Series Models
The world of economics is replete with time-dependent data. Time series models are tailored to tackle this temporal dimension. From analyzing stock prices to forecasting macroeconomic variables, these models are indispensable. Autoregressive Integrated Moving Average (ARIMA) models, for instance, are adept at capturing trends and seasonality in data. In contrast, Vector Autoregression (VAR) models are invaluable for studying the dynamics of multiple interrelated time series variables.
Panel Data Models
Panel data models amalgamate time series and cross-sectional data, yielding a rich dataset that empowers researchers to unravel complex economic relationships. These models permit the exploration of individual heterogeneity, time effects, and cross-sectional correlations simultaneously. The Fixed Effects (FE) and Random Effects (RE) models offer distinct approaches to account for unobserved individual effects and temporal dynamics, thereby providing valuable insights into various economic phenomena, from firm-level productivity to labour market dynamics.
Instrumental Variable (IV) Models
Endogeneity, a common issue in econometric analysis, arises when independent variables are correlated with the error term. IV models come to the rescue by employing instrument variables related to the endogenous variable but not the error term. These models are essential for estimating causal relationships in the presence of endogeneity. For instance, in studying the impact of education on earnings, IV models can use an instrument like compulsory schooling laws to address potential endogeneity in the education variable.
Structural Equation Models (SEM)
SEMs provide a robust framework for understanding complex relationships when dealing with latent constructs and multiple observed variables. These models combine elements of econometrics and psychometrics, making them indispensable in areas like consumer behaviour analysis, measuring intangible concepts like economic well-being, and analyzing the impact of latent variables on observed outcomes.
Bayesian Econometrics
In recent years, Bayesian econometrics has gained prominence, offering a probabilistic framework to estimate parameters and make predictions. Bayesian models incorporate prior beliefs about parameters and update them based on observed data, allowing for a more flexible and coherent approach to econometric modelling. Bayesian methods are particularly beneficial when dealing with limited data or incorporating expert knowledge into the modelling process.
Machine Learning in Econometrics
The fusion of machine learning techniques with econometrics has opened up new avenues for modelling and prediction. Algorithms like neural networks, random forests, and support vector machines can handle vast datasets and complex nonlinear relationships, making them suitable for forecasting economic indicators, stock market movements, and credit risk assessment.
In conclusion, econometrics is a multifaceted landscape adorned with various models tailored to address the diverse and complex economic questions we encounter. Linear regression models, time series models, panel data models, instrumental variable models, structural equation models, Bayesian econometrics, and machine learning techniques collectively form a toolkit that equips economists with the means to decipher economic phenomena, make informed decisions, and shape the future of economies worldwide. Each of these models, with its unique strengths and applications, contributes to the ever-evolving tapestry of econometric analysis, enriching our understanding of the economic world.
Last Update: Sept. 14, 2023, 4:47 p.m.