Linear Regression: A Journey into Predictive Modeling
Post Date:
Analysis Data Models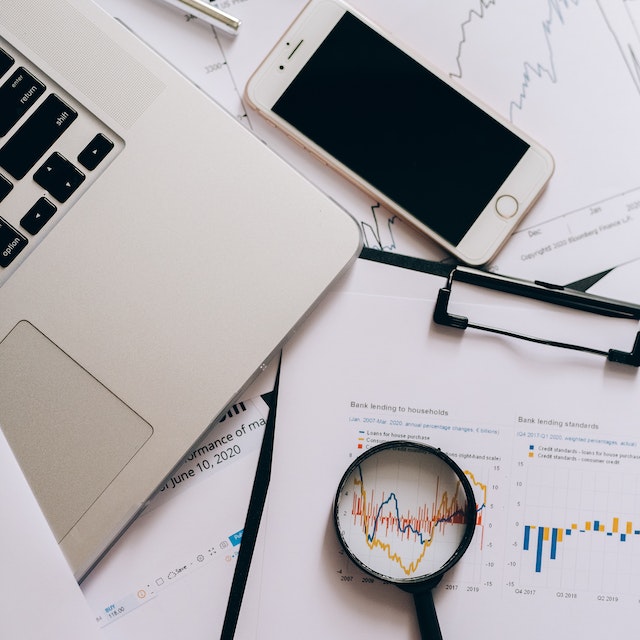
In the vast landscape of data science and machine learning, linear regression is a foundational cornerstone. Its simplicity belies its effectiveness, making it one of the most widely used and cherished tools for predictive modelling. Linear regression models are, in essence, a means of quantifying and understanding the relationships between variables. Whether you are an aspiring data scientist, a seasoned analyst, or simply someone curious about prediction, this journey into linear regression promises to be enlightening and captivating.
At its core, linear regression seeks to establish a linear relationship between a dependent variable and one or more independent variables. This relationship is expressed through a mathematical equation that embodies the principle of least squares, aiming to minimize the sum of squared differences between observed data points and predicted values. The result is a linear equation line that best fits the data, allowing us to make informed predictions based on this fitted model.
The appeal of linear regression is undeniable. Its simplicity makes it accessible to individuals with varying mathematical expertise, while its versatility ensures its relevance in many fields. Imagine predicting housing prices based on square footage, understanding the impact of advertising expenditures on sales, or even forecasting the weather's effect on ice cream sales—linear regression can easily tackle these problems.
The heart of linear regression is its ability to provide valuable insights into the relationships between variables. Through the coefficients of the linear equation, we can decipher which independent variables exert the most influence on the dependent variable and to what extent. This information is helpful in making predictions and understanding the driving forces behind the data. It enables us to identify patterns, outliers, and potential areas for optimization, ultimately contributing to more informed decision-making.
Linear regression comes in various forms, each tailored to specific scenarios. Simple linear regression involves just one independent variable, while multiple linear regression accommodates several predictors. Additionally, there are specialized adaptations like logistic regression for classification problems and polynomial regression for nonlinear relationships. These diverse tools expand the reach of linear regression into a wide array of applications, from business analytics to scientific research.
Furthermore, the advent of technology and sophisticated software packages has democratized the application of linear regression. Gone are the days of manually crunching numbers; now, with the click of a button, one can fit intricate regression models to their data, visualize results, and assess the model's performance. This accessibility empowers individuals from various disciplines to harness the predictive power of linear regression.
In conclusion, linear regression is not merely a statistical technique but a gateway to understanding and harnessing the predictive potential hidden within data. Its simplicity, versatility, and interpretability make it an indispensable tool in the modern era of data-driven decision-making. As we explore linear regression, we will delve deeper into its principles, applications, and best practices. Together, we will unlock the secrets that lie beneath the surface of this unassuming yet potent predictive modelling technique.
Last Update: Dec. 9, 2024, 11:21 p.m.