The Art and Science of Economic Prediction
Post Date:
Analysis Data Models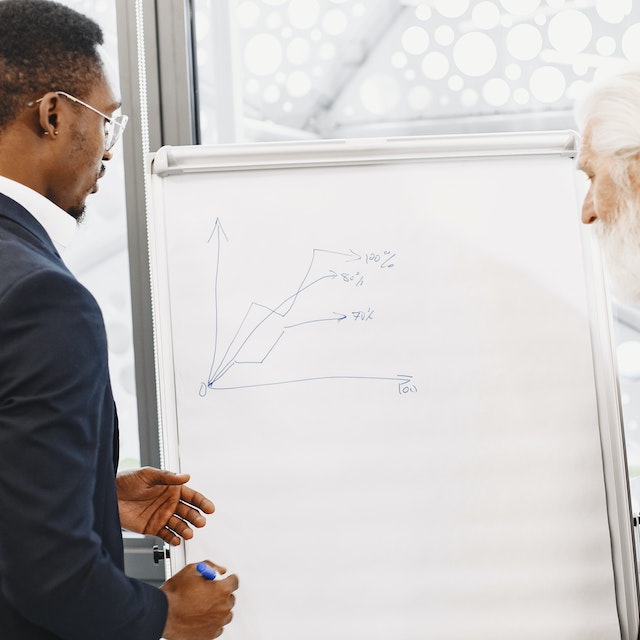
Economic forecasting is crucial in policymakers, businesses, and investors' arsenal. It offers the potential to anticipate economic trends, make informed decisions, and adapt strategies in an ever-changing world. Among the myriad approaches to forecasting, econometrics has emerged as a powerful and versatile method, seamlessly blending economics with mathematics and statistics. This essay delves into the fascinating forecasting world using econometrics, highlighting its significance, principles, challenges, and applications.
Econometrics, at its core, is the art and science of applying statistical techniques to economic data. Doing so aims to uncover patterns, relationships, and trends that may otherwise remain hidden. The primary goal is to use these insights to make predictions, test economic theories, and inform decision-making.
A fundamental principle of econometrics is using mathematical models to represent economic phenomena. These models are constructed using historical data and economic theory. For instance, one might develop a model to forecast inflation based on factors such as interest rates, GDP growth, and money supply. Econometricians then use statistical methods to estimate the model's parameters, test its validity, and, ultimately, make predictions about future economic conditions.
Data plays a pivotal role in the realm of econometrics. With high-quality, reliable data, the accuracy of forecasts is maintained. Economists rely on various data sources, such as government reports, financial statements, and surveys. However, collecting and processing data can be daunting, often requiring rigorous quality control measures to ensure accuracy.
Furthermore, econometricians must grapple with the challenge of handling vast datasets. The advent of big data has ushered in a new era in econometrics, offering the opportunity to analyze more comprehensive and granular information. However, managing and processing such extensive datasets necessitates advanced computational techniques and algorithms.
Econometric models come in various forms, each tailored to specific forecasting objectives. Time series models, for instance, are well-suited to predict economic variables that exhibit a temporal pattern, like stock prices or GDP growth. These models use past observations to make predictions, assuming the future will resemble the past.
In contrast, structural models attempt to understand the underlying economic mechanisms. These models are often more complex and require a deeper understanding of economic theory. They aim to capture the cause-and-effect relationships between variables and can provide valuable insights into the economic forces driving forecasts.
Despite its power and versatility, econometrics has challenges and limitations. Forecasting economic outcomes is inherently uncertain due to the complexity and dynamism of financial systems. As such, econometric models can only provide probabilistic forecasts, acknowledging the inherent uncertainty of economic phenomena.
Econometrics also faces the problem of model misspecification. The accuracy of forecasts depends heavily on the model choice and the underlying assumptions' quality. If the model accurately captures the actual relationships in the data, the estimates can be wildly off the mark.
Econometrics is applied in many fields, from macroeconomic policy analysis to financial market predictions. Central banks use econometric models to guide their decisions on interest rates and monetary policy. Businesses rely on forecasting to make production and investment decisions. Investors use econometric models to allocate their portfolios intelligently.
Econometrics is the art and science of economic prediction, offering a potent blend of mathematics, statistics, and economic theory. Using mathematical models, econometricians analyze historical data to forecast future economic trends, providing valuable insights for policymakers, businesses, and investors. While econometrics is a powerful tool, it has challenges and limitations, notably the uncertainty inherent in economic forecasting. Nevertheless, its continued evolution and integration of big data offer exciting prospects for improving the accuracy and reliability of economic predictions, making it an indispensable tool in an increasingly complex and interconnected world.
Last Update: Sept. 14, 2023, 4:44 p.m.