The Crucial Role of Cleaning and Transforming Data Sets
Post Date:
Coding Data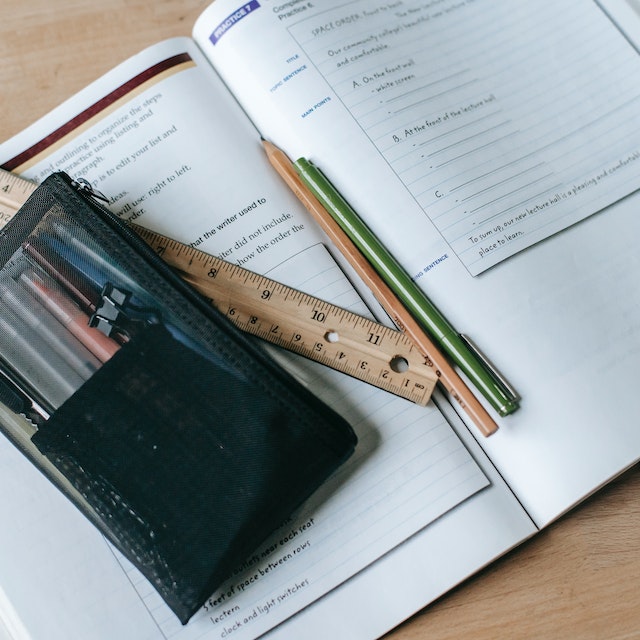
In today's data-driven world, the value and impact of high-quality data cannot be overstated. However, raw data, often riddled with imperfections and inconsistencies, poses significant challenges to deriving meaningful insights. This is where the critical processes of cleaning and transforming data sets come into play. In this essay, we will explore the importance of these processes, highlighting how they enhance data reliability, accuracy, and usability, ultimately leading to more informed decision-making and improved outcomes.
Cleaning and transforming a data set is essential in ensuring the data's reliability and integrity. Raw data often contains errors, missing values, outliers, and inconsistencies due to human entry mistakes, system glitches, or data integration issues. By thoroughly cleaning the data, such errors and inconsistencies can be identified and rectified, increasing the overall reliability of the data set. This, in turn, instils confidence in the data's accuracy and enables stakeholders to trust its insights.
Transforming a data set involves refining and standardizing it, making it consistent and accurate. This includes tasks such as correcting formatting issues, standardizing units of measurement, and normalizing data across different sources. Applying these transformations makes the data more consistent, eliminating variations that could lead to misinterpretations or biased conclusions. Improved accuracy ensures that decisions and analyses are based on reliable information, reducing potential errors and mitigating risks.
Cleaning and transforming a data set is crucial for enabling practical analysis and extracting valuable insights. Analysts can work with complete and accurate data by addressing missing values, outliers, and errors, avoiding skewed results or erroneous conclusions. Moreover, data transformations, such as aggregating or summarizing data, allow for higher-level analysis and pattern identification. Cleaned and transformed data sets provide a solid foundation for applying statistical techniques, data mining algorithms, and machine learning models, leading to more robust and meaningful analysis outcomes.
Decision-making processes heavily rely on accurate and reliable data. By cleaning and transforming data sets, decision-makers gain access to high-quality information, facilitating informed and data-driven decision-making. Cleaned data eliminates biases and ensures that decisions are based on the most accurate representation of reality. Moreover, transformed data sets allow for comprehensive analysis, uncovering hidden trends, patterns, and correlations that may not be apparent in raw data. This empowers decision-makers to make more strategic and practical choices, improving outcomes and performance.
Cleaning and transforming data sets are crucial for integrating and collaborating across different sources and stakeholders. When multiple datasets must be combined or compared, ensuring data consistency, formatting, and accuracy becomes paramount. By cleaning and transforming data, the interoperability and compatibility of different datasets are enhanced, fostering seamless integration and collaboration. This enables organizations to harness the power of diverse data sources, leverage collective intelligence, and drive innovation.
Cleaning and transforming data sets are indispensable processes in data analysis and decision-making. They lay the foundation for reliable, accurate, and meaningful insights, empowering organizations and individuals to make informed choices. By eliminating errors, addressing inconsistencies, and standardizing data, the value and usability of the data are significantly enhanced. Recognizing the importance of cleaning and transforming data sets is critical to unlocking the true potential of data-driven approaches and driving success in the data-driven era.
Last Update: July 18, 2023, 2:35 p.m.